‘Architectural Tricks for Deep Learning in Remote Photoplethysmography’ — a report from the SketchAR team at ICCV 2019, Seoul Korea.
This November, the SketchAR team attended one of the leading Computer Vision conferences in the world — ICCV (IEEE International Conference of Computer Vision).
The conference brought together the best researchers all over the world. It was more than 1000 scientific papers presented, including breakthroughs methods on dozens of rapidly developed fields such as Generative Adversarial Nets (GANs), Domain Adaptation, Zero-shot learning, and others.
SketchAR developers Mikhail Kopeliovich and Mikhail Petrushan presented their paper on camera-based heart rate estimation, “Architectural Tricks for Deep Learning in Remote Photoplethysmography.” They suggested advanced techniques that can be integrated into deep learning architectures, increasing their accuracy.
Abstract
Architectural improvements are studied for convolutional network performing estimation of heart rate (HR) values on color signal patches. Color signals are time series of color components averaged over facial regions recorded by webcams in two scenarios: Stationary (without motion of a person) and Mixed Motion (different motion patterns of a person). HR estimation problem is addressed as a classification task, where classes correspond to different heart rate values within the admissible range of [40; 125] bpm. Both are adding convolutional filtering layers after fully connected layers and involving combined loss function. The first component is a cross-entropy, and second is a squared error between the network output and smoothed one-hot vector, lead to better performance of the HR estimation model in Stationary and Mixed Motion scenarios.
Learn more about the approach here.
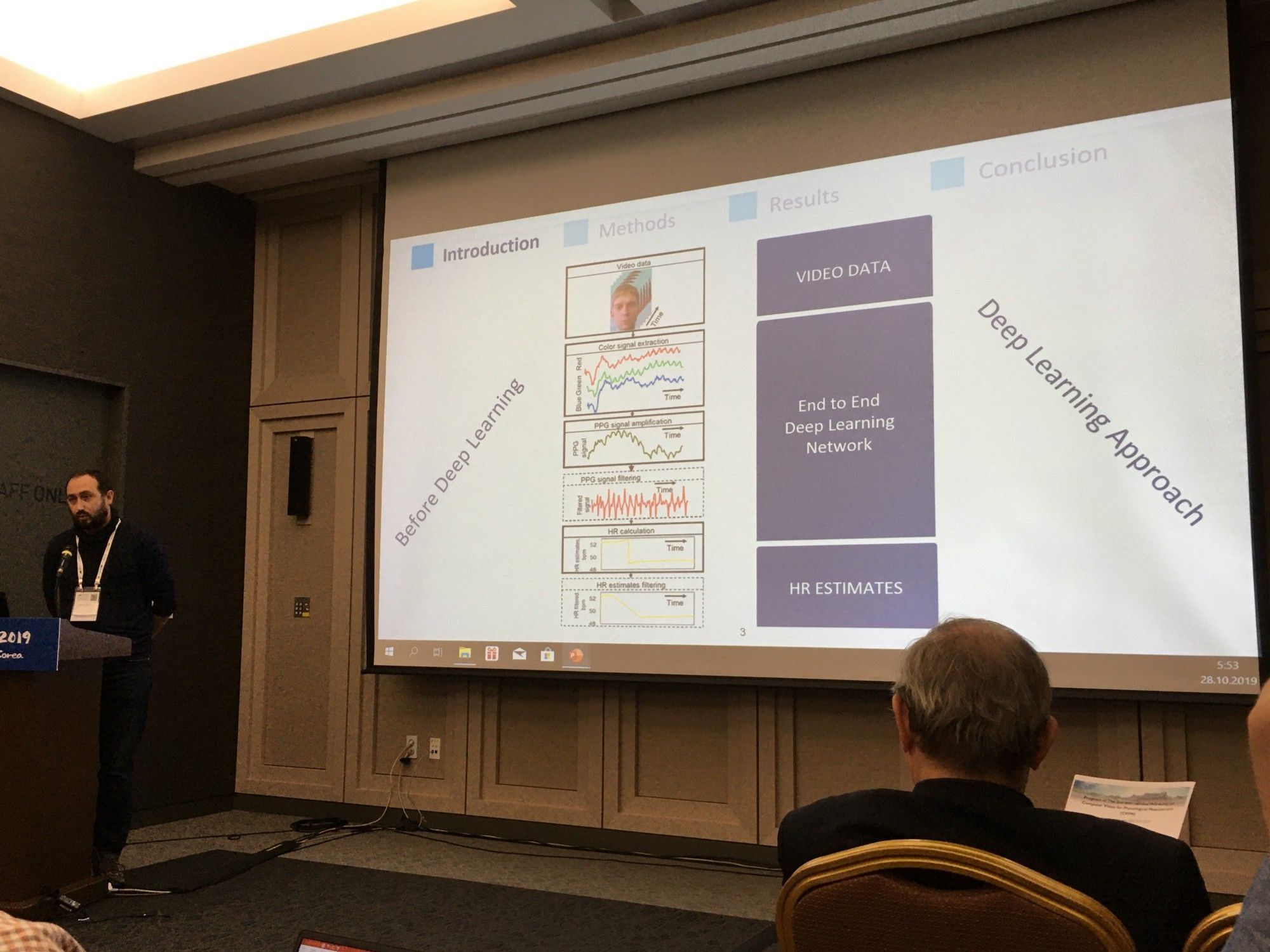
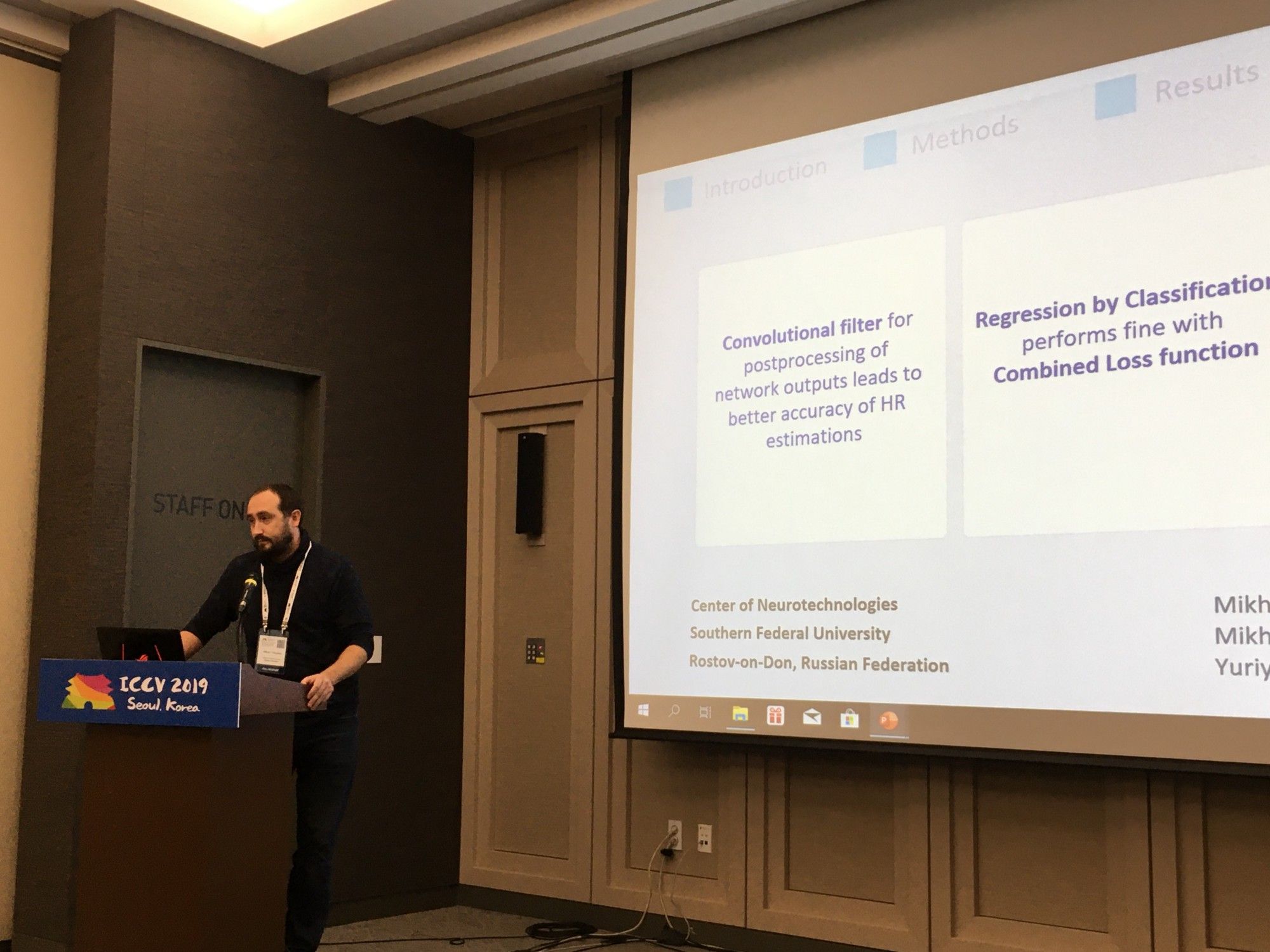
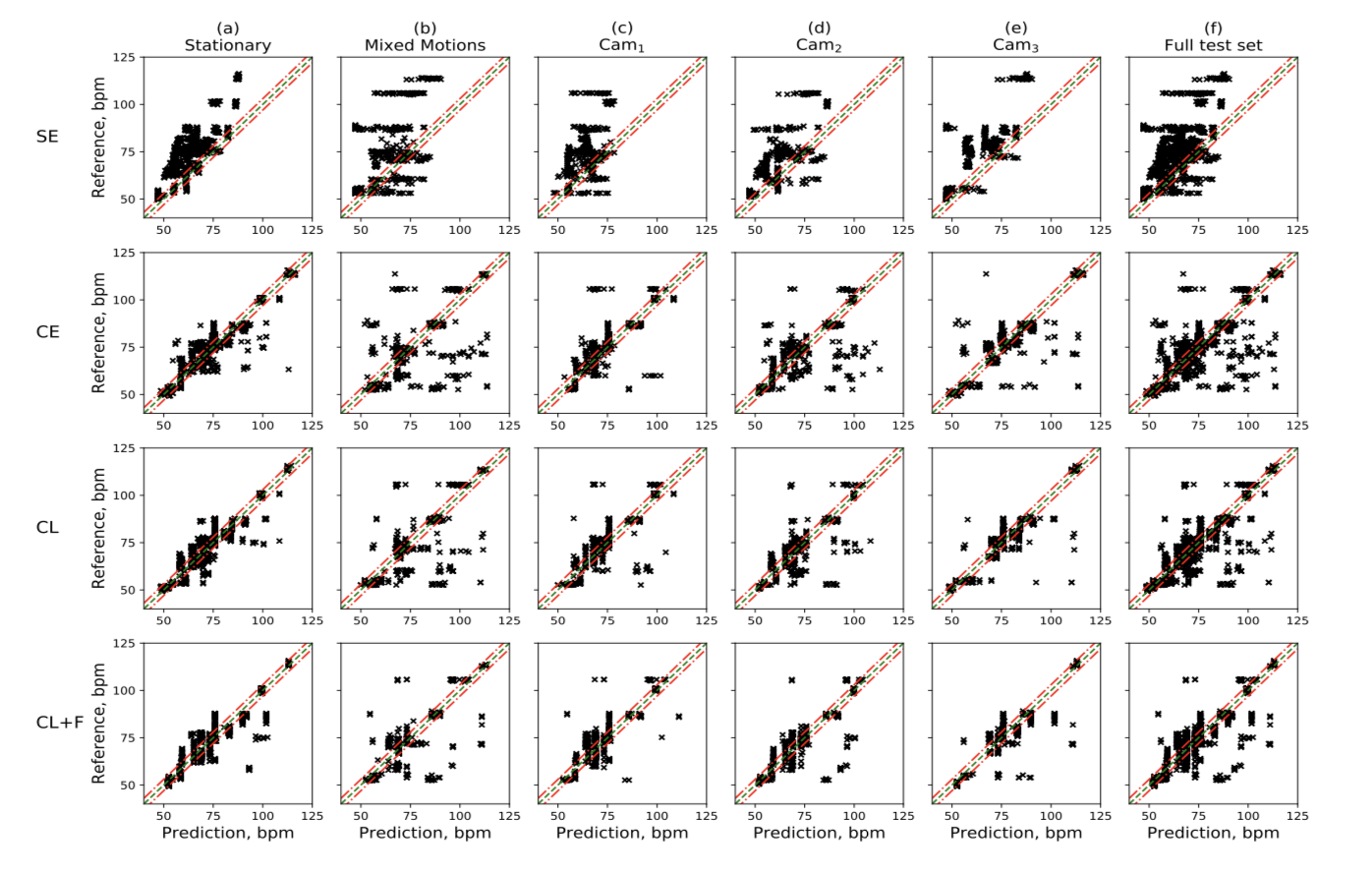
Fill free to drop us a line at hi(a)sketchar.tech
Your,
SketchAR team